The rapid development of computer vision algorithms increasingly allows automatic visual recognition to be incorporated into a suite of emerging applications. Some of these applications have less-than-ideal circumstances such as low-visibility environments, causing image captures to have degradations. In other more extreme applications, such as imagers for flexible wearables, smart clothing sensors, ultra-thin headset cameras, implantable in vivo imaging, and others, standard camera systems cannot even be deployed, requiring new types of imaging devices. Computational photography addresses the concerns above by designing new computational techniques and incorporating them into the image capture and formation pipeline. This raises a set of new questions. For example, what is the current state-of-the-art for image restoration for images captured in non-ideal circumstances? How can inference be performed on novel kinds of computational photography devices?
Continuing the success of the 1st (CVPR'18), 2nd (CVPR'19), and 3rd (CVPR'20) UG2 Prize Challenge workshops, we provide its 4th version for CVPR 2021. It will inherit the successful benchmark dataset, platform and evaluation tools used by the first three UG2 workshops, but will also look at brand new aspects of the overall problem, significantly augmenting its existing scope.
Original high-quality contributions are solicited on the following topics:
- Novel algorithms for robust object detection, segmentation or recognition on outdoor mobility platforms (UAVs, gliders, autonomous cars, outdoor robots etc.), under real-world adverse conditions and image degradations (haze, rain, snow, hail, dust, underwater, low-illumination, low resolution, etc.)
- Novel models and theories for explaining, quantifying, and optimizing the mutual influence between the low-level computational photography tasks and various high-level computer vision tasks, and for the underlying degradation and recovery processes, of real-world images going through complicated adverse visual conditions.
- Novel evaluation methods and metrics for image restoration and enhancement algorithms, with a particular emphasis on no-reference metrics, since for most real outdoor images with adverse visual conditions it is hard to obtain any clean “ground truth” to compare with.
Sponsors

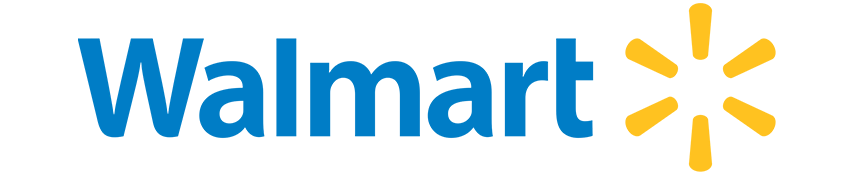
Challenge Categories
Winners
$K
Awarded in prizes
Keynote speakers
Available Challenges
What is the current state-of-the-art for image restoration for images captured in non-ideal circumstances? How can inference be performed on novel kinds of computational photography devices?
The UG2+ Challenge seeks to advance the analysis of "difficult" imagery by applying image restoration and enhancement algorithms to improve analysis performance. Participants are tasked with developing novel algorithms to improve the analysis of imagery captured under problematic conditions.
Object Detection in Poor Visibility Environments
While most current vision systems are designed to perform in environments where the subjects are well observable without (significant) attenuation or alteration, a dependable vision system must reckon with the entire spectrum of complex unconstrained and dynamic degraded outdoor environments. It is highly desirable to study to what extent, and in what sense, such challenging visual conditions can be coped with, for the goal of achieving robust visual sensing.
Sub-Challenges
- Object Detection in the Hazy Condition
- Face Detection in the Low-Light Condition
Action Recognition from Dark Videos
Action recognition has been applied in various fields, yet most current action recognition methods focus on videos shot under normal illumination. In the meantime, there are various scenarios where videos shot under adverse illumination is unavoidable, such as night surveillance, and self-driving at night. It is therefore highly desirable to explore possible methods that are robust and could cope with these scenarios. It would be event better if such methods could utilize web videos, which are widely available and normally shot under normal illumination.
Sub-Challenges
- Fully Supervised Action Recognition in the Dark
- Semi-supervised Action Recognition in the Dark
Keynote speakers
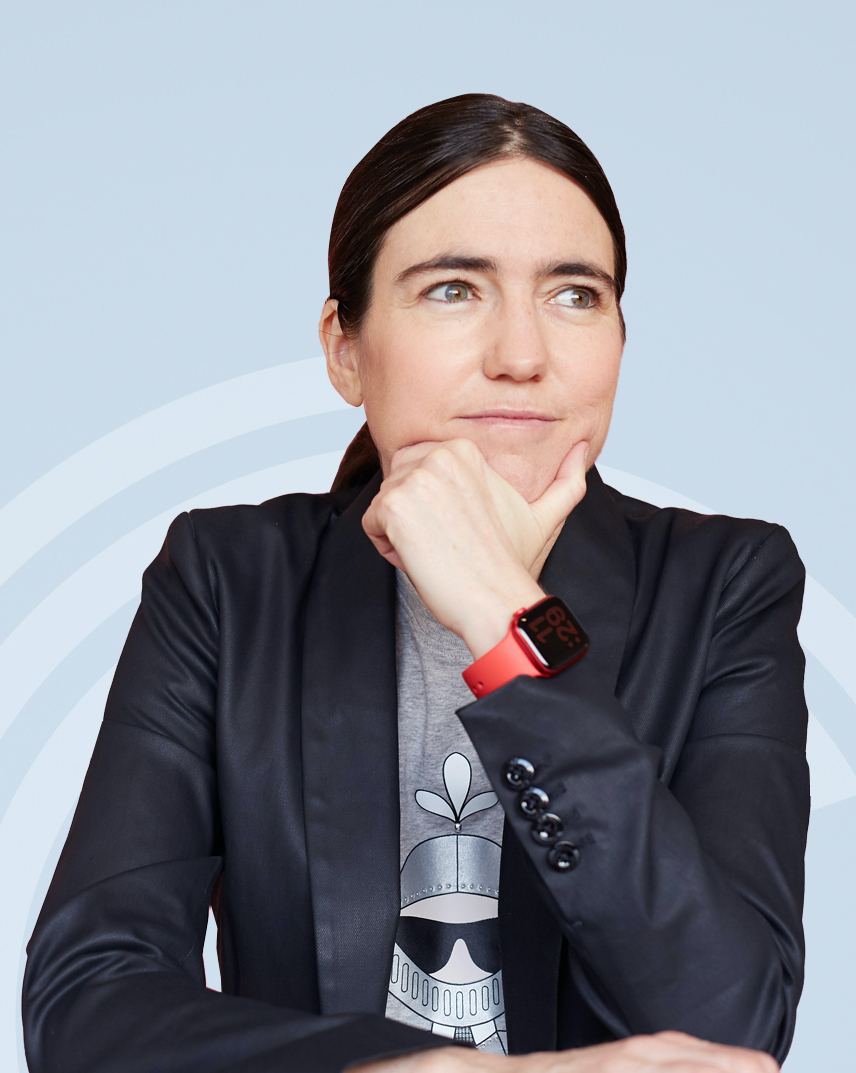
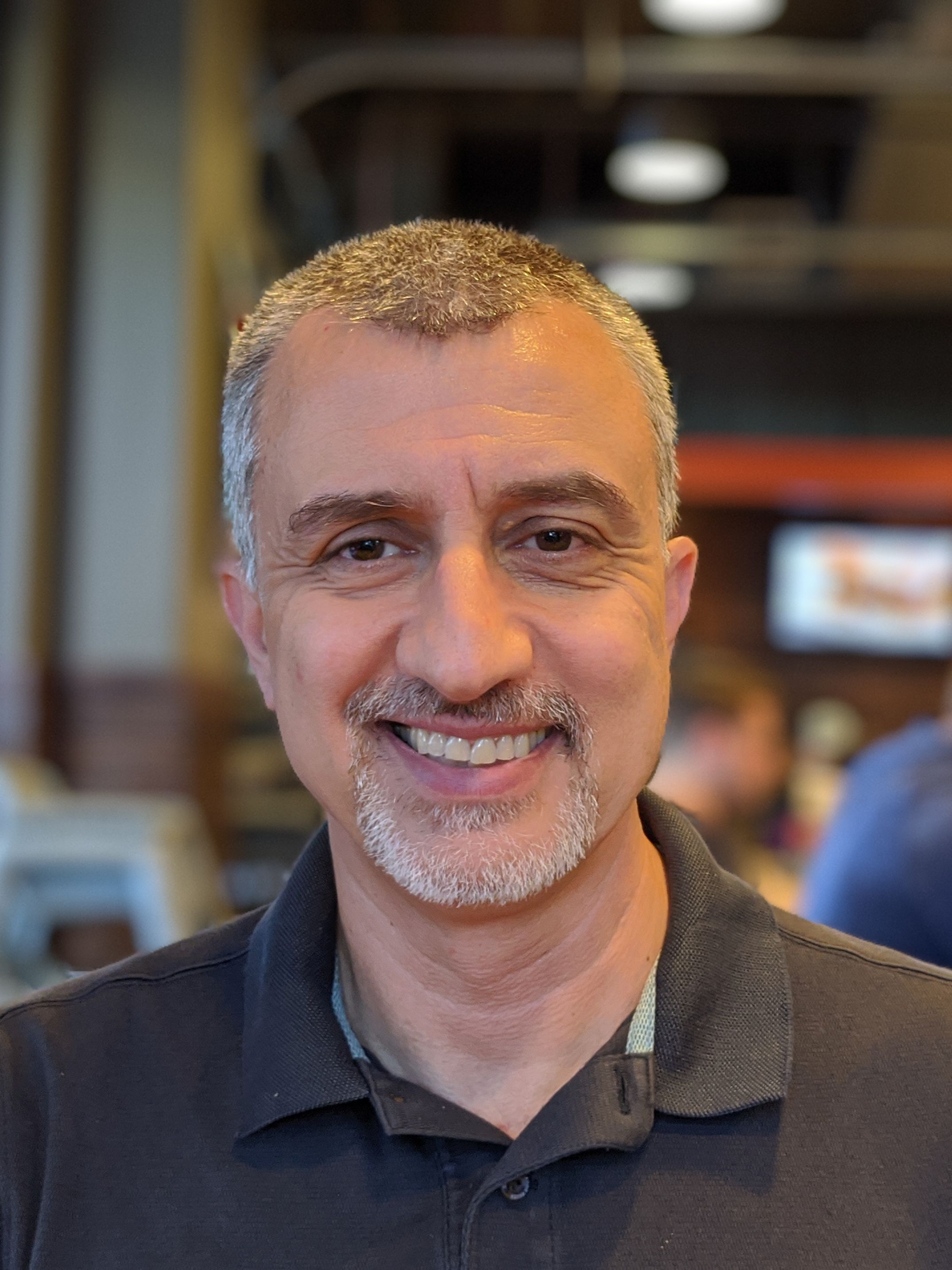
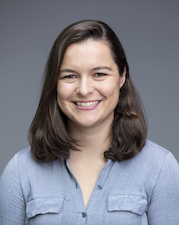
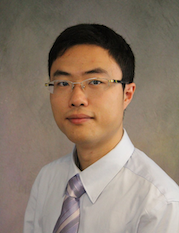
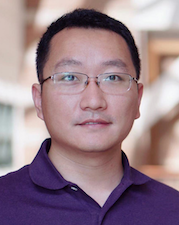
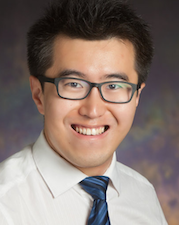
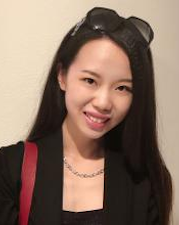
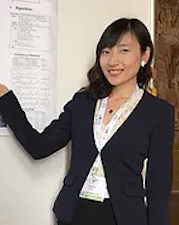
Important Dates
Challenge Registration
January 15 - May 1, 2021
Challenge Dry-run
January 15 - May 1, 2021
Paper Submission Deadline
April 5, 2021
Notification of Paper Acceptance
April 12, 2021
Paper Camera Ready
April 16, 2021
Challenge Final Result Submission
April 30 - May 1, 2021
Challenge Winners Announcement
May 20, 2021
CVPR Workshop
June 19, 2021
Organizers
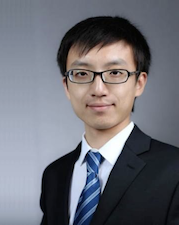
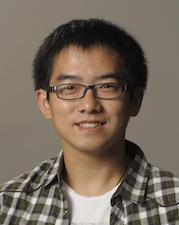
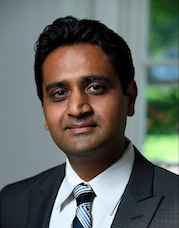
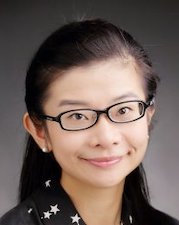
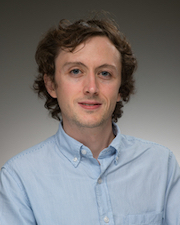
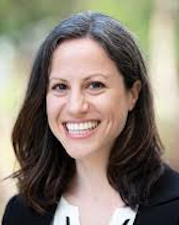
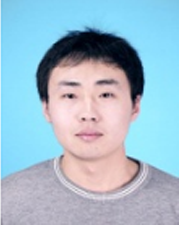
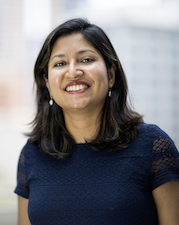
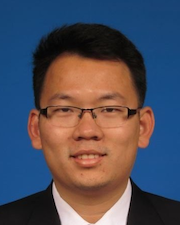
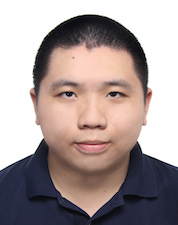
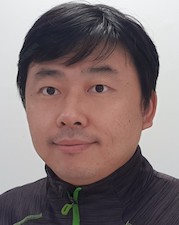