Track 5: UAV Tracking and Pose-estimation
Register for this track
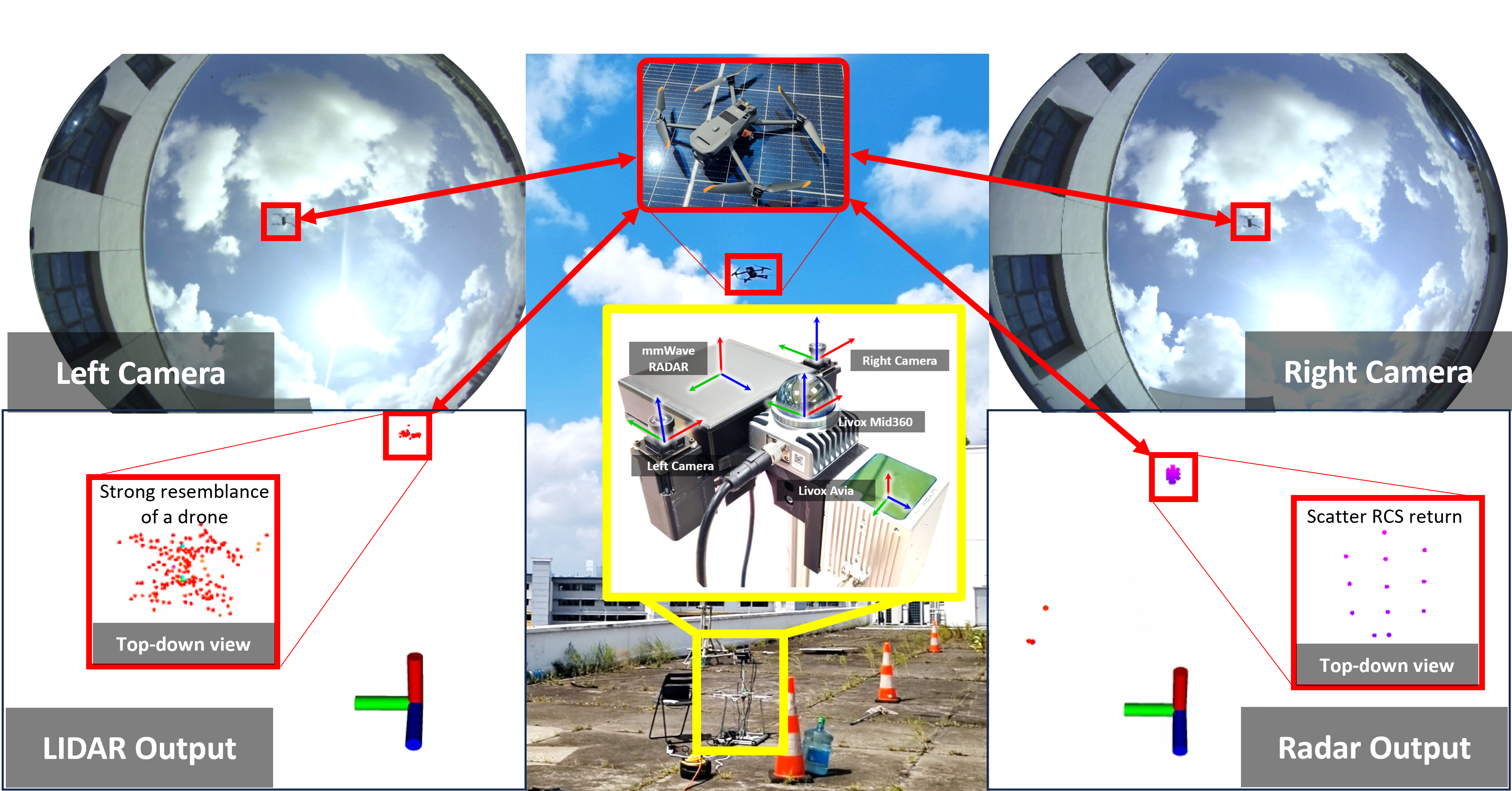
Unmanned aerial vehicles (UAVs), commonly referred to as drones, have become increasingly accessible and played an important role in various fields, such as transportation, photography, search and rescue, bring great benefits to the general public. However, the proliferation and capabilities of small commercial UAVs have also introduced multifaceted security challenges that extend beyond conventional concerns.
Over the past few years, there has been a remarkable rise of research interest with regards to anti-UAV system. Current anti-UAV solutions mainly use visual, radar, and radio frequency (RF) modalities. However, the identification of drones poses a significant challenge for sensors, such as cameras, particularly when drones operate at high altitudes or encounter extreme visual conditions. Therefore, these methods struggle to detect small drones due to their compact size, resulting in a reduced Radar Cross Section and smaller visual presence. Most importantly, the primary focus of the contemporary anti-UAV research is on object detection and 2D tracking, while neglecting the crucial integration of 3D trajectory estimation. This limitation significantly restricts the practical applications of anti-UAV systems in real-world.
Hence, this challenge is designed with the objective of integrating features from diverse modalities to achieve robust 3D UAV position estimation, even in challenging conditions where certain sensors may fail to acquire valid information. This challenge specifically entails the utilization of fisheye camera images, millimeter-wave radar data, and lidar data obtained from a Livox Mid360 and a Livox Avia for both drone type classification and 3D position estimation task, with ground truth provided by a Leica Nova MS60 Multi-Station.
The evaluation process will be conducted on a hold-out set of multimodal datasets derived from the MMAUD dataset, the first dataset dedicated to predicting the 3D position using multimodal data. Participants are required to utilize the provided test data to infer both the position and the type of the drone at the given timestamps and upload a .csv file following a specific format (including specific content and naming format) as specified in this README file.
The ranking criteria of this challenge will be based on i) Mean Square Loss (MSE Loss) with respect to the ground truth label of the testing set and ii) the Classification Accuracy of the type of UAVs of the testing set.
Organizing Committee:
Yuecong Xu (National University of Singapore)
Shenghai Yuan (Nanyang Technological University, Singapore)
Jianfei Yang (Nanyang Technological University, Singapore)
Yizhuo Yang (Nanyang Technological University, Singapore)
Xinhang Xu (Nanyang Technological University, Singapore)
Thien-Minh Nguyen (Nanyang Technological University, Singapore)
Tongxing Jin (Nanyang Technological University, Singapore)
We would like to acknowledge the support from Nanyang Technological University, Singapore and National University of Singapore over the course of this Challenge as well as the construction of the MMAUD dataset. We would also like to specially thank Mr Tan Rong Sheng Zavier Jan and Mr Lee Dong Sheng from Avetics PTE LTD for the clearance of the legal documents and flying the drones.
References:
[1] Yuan S, Yang Y, Nguyen T H, et al. MMAUD: A Comprehensive Multi-Modal Anti-UAV Dataset for Modern Miniature Drone Threats[J]. arXiv preprint arXiv:2402.03706, 2024.
Footer